Table of Contents
As businesses navigate the complexities of supply chains in 2024, the role of AI for Inventory Management has become indispensable. By leveraging AI, organizations are achieving real-time insights, optimizing stock levels, and responding faster to market demands. AI’s capabilities go beyond traditional methods, offering automation, predictive analytics, and decision-making tools that transform how inventory is managed.
This article explores powerful techniques of AI for Inventory Management, revealing how businesses can enhance efficiency, reduce costs, and make better inventory decisions in today’s digital landscape.
1. Demand Forecasting with AI Algorithms
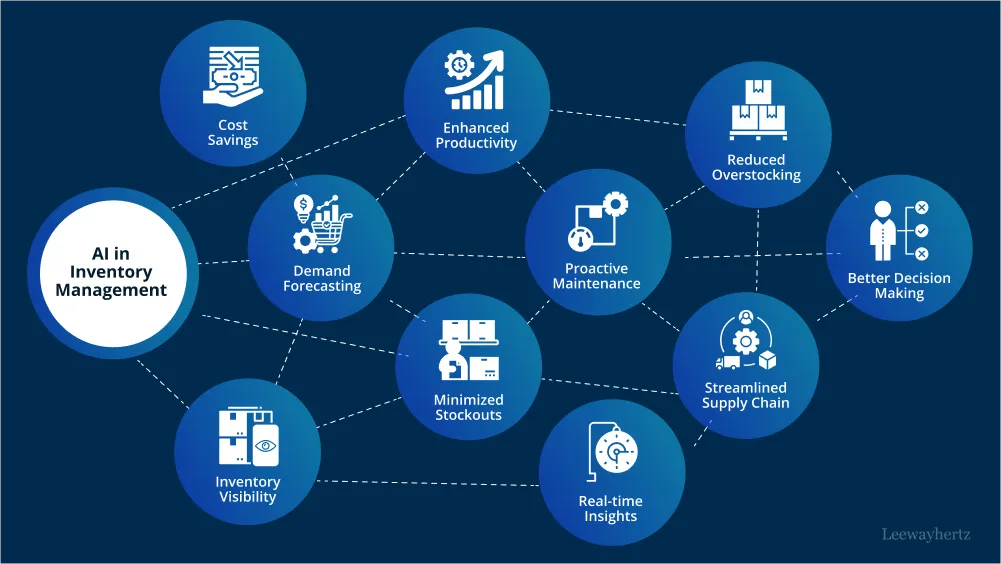
AI for Inventory Management is revolutionizing demand forecasting by using advanced algorithms to analyze large data sets. Unlike manual forecasting, AI algorithms consider multiple variables such as seasonal trends, economic indicators, and historical data to predict demand accurately. These AI-driven insights enable companies to adjust their inventory levels, reducing the risk of overstocking or stockouts.
By anticipating demand accurately, businesses can plan better, align supply with demand, and avoid unnecessary holding costs.
2. Automated Inventory Tracking and Monitoring
Automation is another powerful technique in AI for Inventory Management. Traditionally, tracking inventory involves time-consuming manual processes, but AI simplifies this by integrating with IoT devices and RFID tags. AI-powered systems continuously monitor inventory levels in real time, ensuring that the stock count is always accurate.
Automated inventory tracking improves visibility, allowing businesses to identify discrepancies immediately and prevent losses due to misplaced or stolen goods. This real-time tracking system is critical for companies with extensive or fast-moving inventories, as it helps maintain operational accuracy and efficiency.
3. Predictive Analytics for Supplier Management
Supplier management is a key component of inventory management, and AI for Inventory Management enhances this process by utilizing predictive analytics. By analyzing historical data on supplier performance, reliability, and lead times, AI provides companies with insights to select and manage suppliers more effectively. Predictive analytics enables businesses to foresee potential supply disruptions and mitigate risks by securing alternative suppliers. This proactive approach ensures that inventory levels remain stable, even during supply chain fluctuations.
4. Intelligent Reordering Systems
AI for Inventory Management introduces intelligent reordering systems that automate the process of replenishing stock. By setting parameters based on demand forecasts, sales cycles, and stock thresholds, AI-driven systems can trigger reorders automatically when inventory reaches a specific level. This minimizes human error and ensures that inventory is always at optimal levels. Intelligent reordering is particularly useful in industries with high turnover rates, where delays in restocking could result in significant revenue losses.
5. Enhanced Inventory Classification
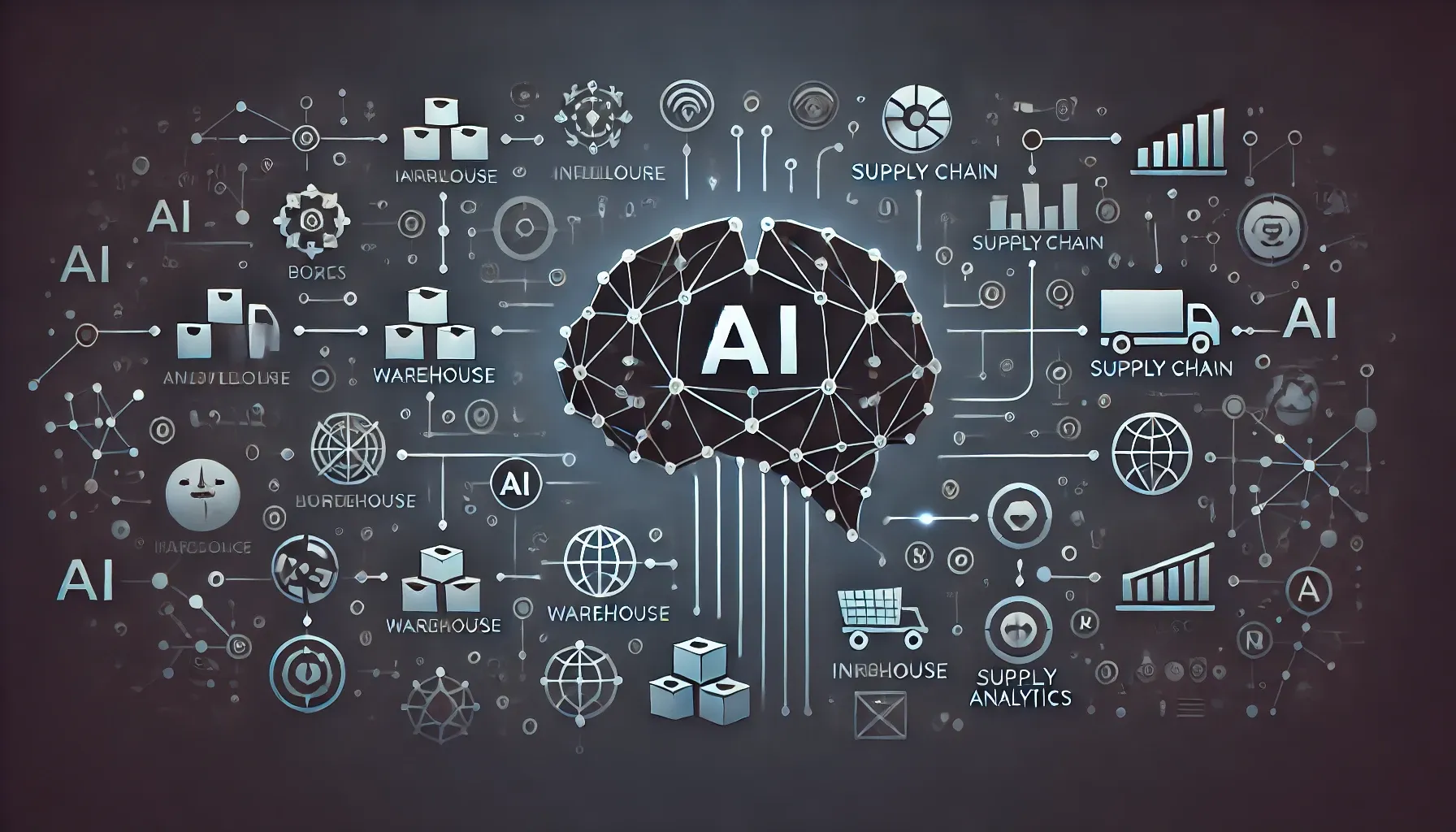
Inventory classification helps businesses prioritize items based on demand, cost, and sales patterns. AI for Inventory Management employs machine learning algorithms to analyze inventory data and categorize items accurately.
For instance, AI can implement the ABC classification method, where A items are high-priority, B items are mid-priority, and C items are low-priority. AI-driven classification allows managers to focus resources on high-demand items, reducing unnecessary stock for less critical products. This optimization technique ultimately lowers holding costs and improves profitability.
6. Dynamic Pricing Models
Dynamic pricing is a powerful tool in AI for Inventory Management that helps companies adjust prices based on current stock levels, demand, and competitive pricing.
By using AI to monitor these factors, companies can increase prices during high demand and lower them when inventory levels are high. This approach enables businesses to manage their inventory effectively while maximizing revenue opportunities. Dynamic pricing also promotes a balanced stock turnover, reducing the likelihood of overstock or unsold products.
7. Reduction of Waste through Expiry Management
For industries handling perishable goods, AI for Inventory Management offers expiry management solutions that reduce waste. By tracking expiry dates and demand, AI helps managers prioritize selling items with near expiration, thus reducing spoilage and waste. AI-driven expiry management ensures that stock rotation is optimal, aligning inventory turnover with product shelf life. This technique is especially beneficial for the food, pharmaceutical, and healthcare industries, where waste reduction directly impacts profitability and compliance.
8. Inventory Optimization with Simulation Models
AI for Inventory Management also employs simulation models to test different inventory strategies. Using data-driven simulations, AI can model various scenarios to predict outcomes, such as demand spikes or supply chain disruptions. These simulations provide decision-makers with insights into the best strategies for inventory management, enabling them to prepare for potential challenges. Inventory optimization through simulation minimizes risks, helping companies maintain continuity and efficiency even during uncertain periods.
9. Risk Assessment and Anomaly Detection
AI for Inventory Management strengthens risk management by identifying potential anomalies in inventory data. Through machine learning, AI systems can detect patterns that indicate fraud, theft, or errors in stock levels. Anomaly detection algorithms provide an additional layer of security by flagging suspicious activities, allowing managers to investigate and prevent losses. This proactive approach reduces the risk of discrepancies and enhances the reliability of inventory data, which is critical for making informed decisions.
10. Integration with Other Business Systems
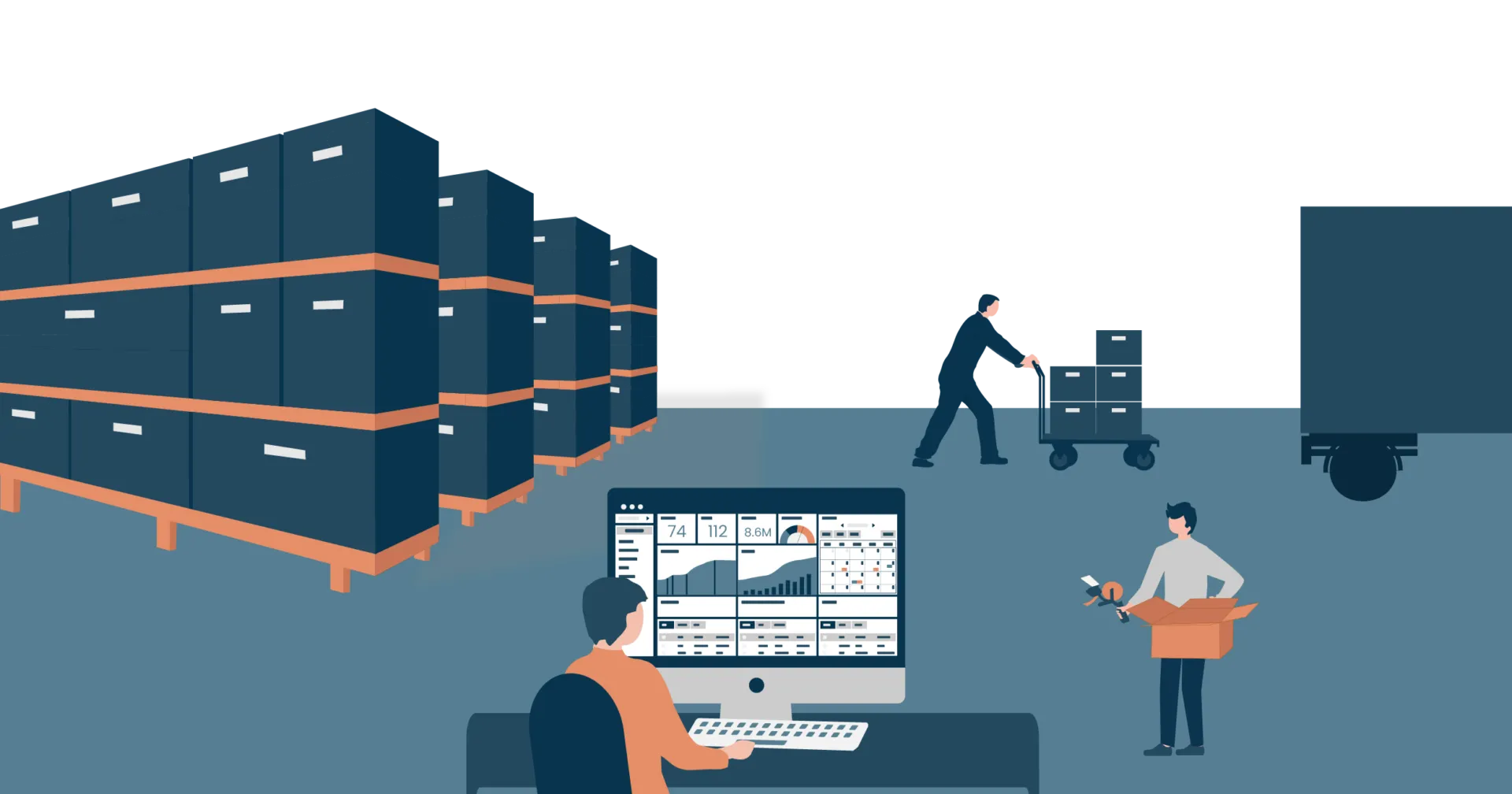
Finally, AI for Inventory Management works best when integrated with other business systems such as ERP, CRM, and sales platforms. By linking inventory management with other core functions, AI creates a seamless flow of information, enhancing data accuracy and decision-making across departments. This integration allows businesses to respond faster to changes in sales trends, customer preferences, or supply chain dynamics, ensuring that inventory management aligns with overall business objectives.
The Future of AI in Inventory Management
AI for Inventory Management is transforming the way businesses manage stock, predict demand, and optimize resources. From predictive analytics to automated tracking and intelligent reordering, AI techniques help companies enhance efficiency, reduce costs, and maintain a balanced stock level. As businesses adopt these powerful techniques, AI for Inventory Management in 2024 is set to play a crucial role in the success of modern supply chains.
By embracing these advancements, companies can stay competitive, meet customer demands, and navigate the challenges of today’s complex market landscape.