Table of Contents
Introduction
Artificial intelligence (AI) is a broad and diverse field that encompasses many subfields and applications. Among them, two of the most prominent and promising ones are generative AI and predictive AI. These two types of AI have different goals, methods, and outcomes, but they also share some commonalities and interactions.
In this blog post, we will explore what generative AI and predictive AI are, how they differ from each other, how they are used in various domains, and what are the future trends and opportunities of these two types of AI. By the end of this blog post, you will have a better understanding of the difference and significance of generative AI and predictive AI, and why they matter for the advancement of AI and humanity.
What is Generative AI?
Generative AI is a type of AI that aims to generate new and original data or content that is similar or related to the existing data or content. For example, generative AI can create realistic images, videos, audio, text, or music that are not present in the original dataset but are consistent with its style, theme, or structure. Generative AI can also modify, enhance, or transform the existing data or content in novel and creative ways.
Some of the most common and popular techniques of generative AI are generative adversarial networks (GANs), variational autoencoders (VAEs), and transformers. GANs are composed of two neural networks: a generator and a discriminator. The generator tries to produce fake data that can fool the discriminator, while the discriminator tries to distinguish between real and fake data.
Through this adversarial process, the generator learns to generate realistic and high-quality data. VAEs are another type of neural network that can learn the latent representation of the data and generate new data by sampling from the latent space. VAEs can also perform various operations on the latent space, such as interpolation, extrapolation, or arithmetic.
Transformers are a type of neural network that can process sequential data, such as text or speech, using attention mechanisms. Transformers can generate coherent and fluent text or speech by learning the context and dependencies of the data.
Generative AI has many advantages and challenges. Some of the advantages are:
- Creativity: Generative AI can produce novel and original data or content that can inspire human creativity and innovation.
- Diversity: Generative AI can generate diverse and varied data or content that can enrich the existing data or content and increase its value and utility.
- Realism: Generative AI can generate realistic and high-quality data or content that can mimic or surpass human perception and performance.
- Scalability: Generative AI can generate large amounts of data or content that can augment or replace the need for human data or content collection and creation.
Some of the challenges are:
- Evaluation: Generative AI is difficult to evaluate objectively and quantitatively, as there are no clear and universal metrics or criteria to measure the quality, diversity, or relevance of the generated data or content.
- Ethics: Generative AI can raise ethical and social issues, such as privacy, security, authenticity, accountability, or fairness, as it can generate fake or misleading data or content that can deceive or harm human users or society.
- Control: Generative AI can be hard to control or regulate, as it can generate unexpected or undesirable data or content that can violate human values, norms, or laws.
What is Predictive AI?
Predictive AI is a type of AI that aims to predict future or unknown outcomes or events based on past or present data or information. For example, predictive AI can forecast the weather, the stock market, customer behavior, or disease progression based on historical or current data or information. Predictive AI can also infer the hidden or missing data or information based on the available or observable data or information.
Some of the most common and popular techniques of predictive AI are regression, classification, clustering, and anomaly detection. Regression is a technique that can predict a continuous or numerical value based on the input variables, such as predicting the house price based on the size, location, or condition of the house.
Classification is a technique that can predict a discrete or categorical value based on the input variables, such as predicting the sentiment of a text based on the words, tone, or context of the text. Clustering is a technique that can group the data or information based on the similarity or dissimilarity of the data or information, such as grouping the customers based on their preferences, behaviors, or demographics.
Anomaly detection is a technique that can identify outliers or abnormal data or information based on the distribution or pattern of the data or information, such as detecting fraud or intrusion based on the deviation or inconsistency of the data or information.
Predictive AI has many advantages and challenges. Some of the advantages are:
- Accuracy: Predictive AI can provide accurate and reliable predictions or inferences that can improve human decision-making and performance.
- Efficiency: Predictive AI can provide fast and timely predictions or inferences that can save human time and resources.
- Interpretability: Predictive AI can provide explainable and understandable predictions or inferences that can increase human trust and confidence.
- Bias: Predictive AI can reduce or eliminate human bias or error that can affect human judgment and action.
Some of the challenges are:
- Data: Predictive AI depends on the quality, quantity, and availability of the data or information, as it can affect the validity, reliability, and generalizability of the predictions or inferences.
- Uncertainty: Predictive AI cannot guarantee the certainty or correctness of the predictions or inferences, as there are always unknown or unpredictable factors or events that can influence the outcomes or events.
- Ethics: Predictive AI can raise ethical and social issues, such as privacy, security, fairness, or accountability, as it can use or disclose sensitive or personal data or information that can affect human rights or interests.
- Control: Predictive AI can be hard to control or regulate, as it can make or influence decisions or actions that can have significant or irreversible consequences for human users or society.
How are Generative AI and Predictive AI Different?
Generative AI and predictive AI are different in many aspects, such as their goals, inputs, outputs, methods, and evaluation metrics. Here are some of the main differences between generative AI and predictive AI:
- Goals: Generative AI aims to generate new and original data or content, while predictive AI aims to predict future or unknown outcomes or events.
- Inputs: Generative AI uses existing or related data or content as inputs, while predictive AI uses past or present data or information as inputs.
- Outputs: Generative AI produces synthetic or artificial data or content as outputs, while predictive AI produces probable or possible outcomes or events as outputs.
- Methods: Generative AI uses generative models, such as GANs, VAEs, or transformers, as methods, while predictive AI uses predictive models, such as regression, classification, clustering, or anomaly detection, as methods.
- Evaluation Metrics: Generative AI uses subjective and qualitative metrics, such as human perception, preference, or satisfaction, as evaluation metrics, while predictive AI uses objective and quantitative metrics, such as accuracy, precision, or recall, as evaluation metrics.
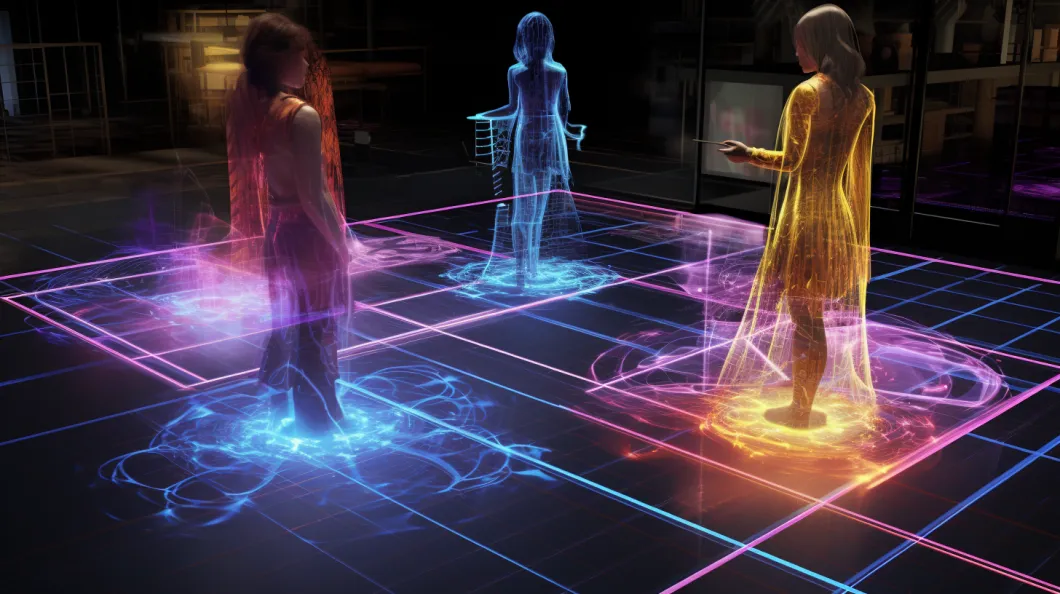
However, generative AI and predictive AI are not completely independent or isolated from each other. They can also complement or compete with each other in some scenarios. Here are some examples of how generative AI and predictive AI can interact with each other:
- Complement: Generative AI can complement predictive AI by providing more or better data or content for predictive AI to use or learn from, such as generating synthetic or augmented data or content that can increase the diversity, quality, or quantity of the data or content. Predictive AI can complement generative AI by providing feedback or guidance for generative AI to improve or optimize its generation process or outcome, such as predicting the quality, diversity, or relevance of the generated data or content.
- Compete: Generative AI can compete with predictive AI by challenging or undermining its prediction or inference ability or validity, such as generating fake or misleading data or content that can deceive or confuse predictive AI. Predictive AI can compete with generative AI by replacing or surpassing its generation ability or creativity, such as predicting or inferring the data or content that can match or exceed the data or content generated by generative AI.
How are Generative AI and Predictive AI Used in Various Domains?
Generative AI and predictive AI have many use cases and applications in various domains, such as art, music, gaming, healthcare, education, business, etc. Here are some examples of how generative AI and predictive AI are used in these domains:
- Art: Generative AI can be used to create or enhance artistic works, such as paintings, drawings, sculptures, or animations, that can express human emotions, ideas, or styles, or generate new and original artistic works that can challenge or inspire human creativity or appreciation. Predictive AI can be used to analyze or evaluate artistic works, such as identifying the artist, genre, or period of the artistic works, or predicting the value, popularity, or impact of the artistic works.
- Music: Generative AI can be used to create or enhance musical works, such as songs, melodies, lyrics, or instruments, that can reflect human moods, tastes, or cultures, or generate new and original musical works that can entertain or educate human listeners or musicians. Predictive AI can be used to analyze or evaluate musical works, such as recognizing the singer, genre, or mood of the musical works, or predicting the quality, diversity, or relevance of the musical works.
- Gaming: Generative AI can be used to create or enhance gaming elements, such as characters, environments, stories, or dialogues, that can provide immersive and engaging gaming experiences, or generate new and original gaming elements that can adapt or respond to the player’s actions or preferences. Predictive AI can be used to analyze or evaluate gaming elements, such as estimating the difficulty, complexity, or fun of the gaming elements, or predicting the behavior, strategy, or outcome of the player or the game.
- Healthcare: Generative AI can be used to create or enhance medical data or information, such as images, signals, reports, or records, that can assist or improve medical diagnosis, treatment, or research, or generate new and original medical data or information that can simulate or augment the existing medical data or information. Predictive AI can be used to analyze or evaluate medical data or information, such as detecting the disease, risk, or progression of the patient, or predicting the outcome, effect, or impact of the medical intervention or policy.
- Education: Generative AI can be used to create or enhance educational content or resources, such as books, courses, quizzes, or feedback, that can support or facilitate learning, teaching, or assessment, or generate new and original educational content or resources that can personalize or customize the learning, teaching, or assessment process or outcome. Predictive AI can be used to analyze or evaluate educational content or resources, such as measuring the quality, difficulty, or relevance of the educational content or resources, or predicting the performance, progress, or potential of the learner or the teacher.
- Business: Generative AI can be used to create or enhance business data or information, such as products, services, marketing, or sales, that can increase or optimize the value, utility, or satisfaction of the business, or generate new and original business data or information that can innovate or diversify the business. Predictive AI can be used to analyze or evaluate business data or information, such as identifying the trends, patterns, or opportunities of the business, or predicting the demand, supply, or profit of the business.
What are the Future Trends and Opportunities of Generative AI and Predictive AI?
Generative AI and predictive AI are constantly evolving and improving, as they are driven by the advances in AI research and development, the availability and accessibility of data and computing resources, and the demands and expectations of human users and society.
Here are some of the future trends and opportunities of generative AI and predictive AI:
- Multimodal: Generative AI and predictive AI will be able to handle and integrate multiple types and modes of data or content, such as text, image, video, audio, or speech, and generate or predict multimodal data or content that can enhance or enrich the information, communication, or interaction of human users or society.
- Cross-domain: Generative AI and predictive AI will be able to transfer and apply their knowledge and skills across different domains, such as art, music, gaming, healthcare, education, or business, and generate or predict cross-domain data or content that can expand or connect the boundaries, possibilities, or opportunities of human users or society.
- Interactive: Generative AI and predictive AI will be able to interact and collaborate with human users or society, and generate or predict interactive data or content that can adapt or respond to the feedback, preferences, or needs of human users or society.
- Collaborative: Generative AI and predictive AI will be able to cooperate and coordinate with each other, and generate or predict collaborative data or content that can complement or enhance the generation or prediction ability or outcome of each other.
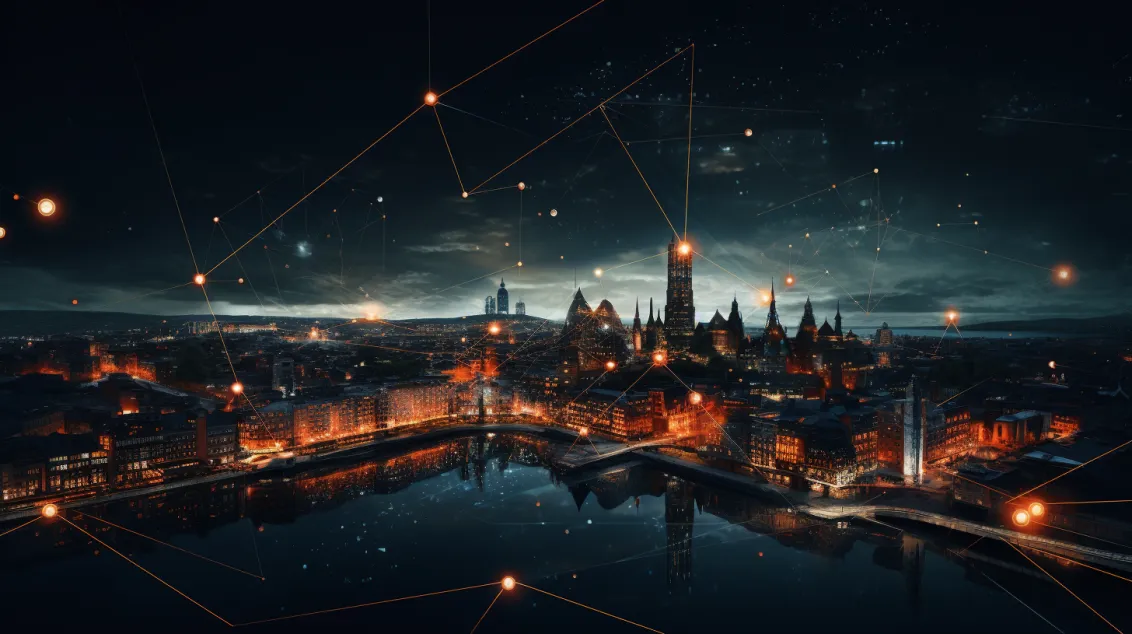
Generative AI and predictive AI will have a significant impact and implications on human users and society, as they will affect various aspects of human life, such as culture, economy, ethics, etc. Here are some of the potential impact and implications of generative AI and predictive AI:
- Culture: Generative AI and predictive AI will influence and shape human culture, as they will create or enhance the cultural products, expressions, or values of human users or society, or generate or predict new and original cultural products, expressions, or values that can challenge or inspire human creativity or appreciation.
- Economy: Generative AI and predictive AI will influence and shape the human economy, as they will create or enhance the economic products, services, or markets of human users or society, or generate or predict new and original economic products, services, or markets that can increase or optimize the value, utility, or satisfaction of human users or society.
- Ethics: Generative AI and predictive AI will influence and shape human ethics, as they will create or enhance the ethical issues, dilemmas, or solutions of human users or society, or generate or predict new and original ethical issues, dilemmas, or solutions that can raise or resolve the moral, social, or legal questions or concerns of human users or society.
Conclusion
In this blog post, we have explored what generative AI and predictive AI are, how they differ from each other, how they are used in various domains, and what are the future trends and opportunities of these two types of AI. We have learned that generative AI and predictive AI have different goals, methods, and outcomes, but they also share some commonalities and interactions.
We have also learned that generative AI and predictive AI have many use cases and applications in various domains and that they have a significant impact and implications on human users and society.
We hope that this blog post has given you a better understanding of the difference and significance of generative AI and predictive AI, and why they matter for the advancement of AI and humanity. Thank you for reading this blog post. We would love to hear your feedback, comments, or questions. Please feel free to share your thoughts with us in the comment section below.