Table of Contents
Artificial Intelligence (AI) has witnessed remarkable advances in recent years, thanks to the power of Large Language Models (LLMs). These models can perform various tasks across different sub-fields of AI, such as Natural Language Processing, Natural Language Generation, and Computer Vision.
However, one of the challenges that remains is how to generate high-quality and coherent new views from limited input, especially for human faces.
A team of researchers from ByteDance has proposed a novel solution to this problem. They have developed DiffPortrait3D, a conditional diffusion model that can transform a single two-dimensional (2D) portrait into a realistic and consistent three-dimensional (3D) representation.
It can handle a wide range of portraits, including those with different expressions, poses, and styles, without requiring any fine-tuning or optimization.
How DiffPortrait3D Works
DiffPortrait3D leverages the generative prior of 2D diffusion models, which are pre-trained on large image datasets, to render new views of a face. It also uses a disentangled attention mechanism to control the appearance and the camera pose of the output. The model takes a reference image as the input and injects its appearance context into the self-attention layers of the frozen UNets, which are part of the diffusion process.
To change the camera pose, it uses a conditional control module that analyzes a condition image of the same subject from the desired angle. This module allows the model to fuse the facial features from different views and produce a coherent 3D representation.
How DiffPortrait3D Improves Visual Consistency
To enhance the quality and realism of the output, DiffPortrait3D also incorporates a cross-view attention module and a 3D-aware noise generation technique. The cross-view attention module helps the model to align the facial landmarks and details across different views, especially when the input has extreme expressions or poses.
The 3D-aware noise generation technique adds noise to the output in a 3D-consistent manner, which improves the robustness and stability of the synthesis.
How DiffPortrait3D Performs
The researchers have evaluated DiffPortrait3D on challenging multi-view and in-the-wild datasets and compared it with existing methods. The results show that it achieves state-of-the-art performance both qualitatively and quantitatively.
The model can generate realistic and high-fidelity 3D portraits from a single image while preserving the identity and expression of the subject. The model can also handle various artistic styles and settings, demonstrating its versatility and zero-shot capability.
What DiffPortrait3D Contributes
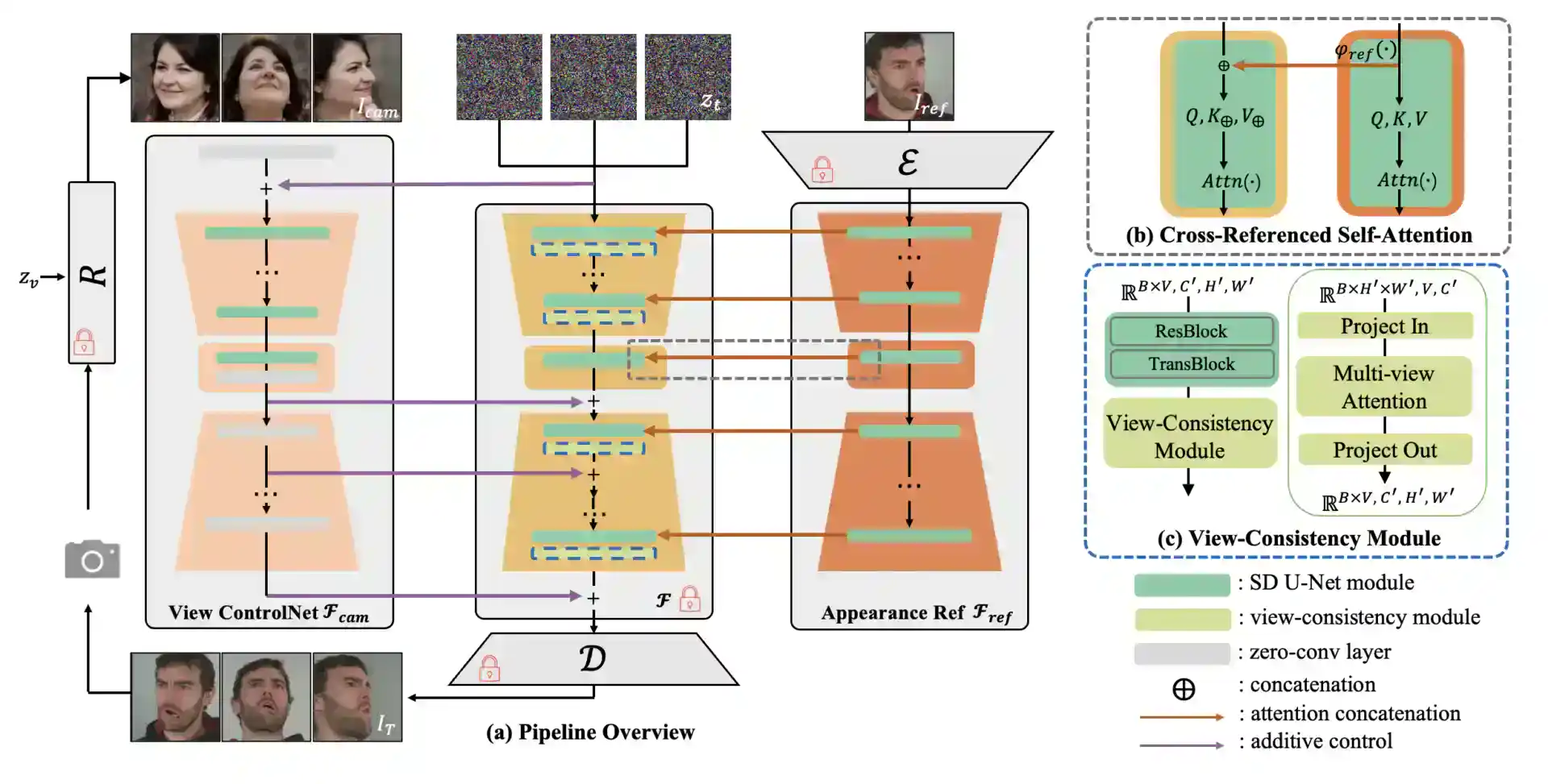
The main contributions are:
- It introduces a novel zero-shot method for 3D portrait synthesis from a single image, by extending 2D diffusion models.
- It demonstrates impressive results in generating novel views of faces, with various appearances, expressions, poses, and style attributes, without needing any fine-tuning.
- It uses a separate control mechanism for appearance and camera pose, which enables flexible and efficient camera manipulation, without affecting the identity or expression of the subject.
- It combines a cross-view attention module and a 3D-aware noise generation technique, which provide long-range consistency and realism in 3D views.
It is a breakthrough in the field of 3D portrait synthesis and opens up new possibilities for applications such as face editing, animation, and virtual reality.
Some other applications
DiffPortrait3D is a novel method for 3D portrait synthesis from a single image, which can generate realistic and consistent views of faces from different angles. Some of the possible applications of it are:
- Face editing: DiffPortrait3D can be used to modify the appearance, expression, pose, and style of a face image, or to create new faces from scratch. For example, one can use it to change the hair color, facial hair, makeup, glasses, or accessories of a face, or to generate a face with different ages, gender, ethnicity, or emotion.
- Animation: It can be used to animate a face image, by generating a sequence of images with different camera poses or expressions. For example, one can use it to create a talking head video, a facial expression recognition system, or a face swap application.
- Virtual reality: DiffPortrait3D can be used to create immersive and interactive virtual environments, by generating 3D portraits of real or fictional characters. For example, one can use it to create a virtual avatar, a digital twin, or a hologram of a person.